Driven by the "double carbon" goal, energy storage technology has become the core carrier of the energy revolution. From electric vehicle batteries to grid-level energy storage plants, the performance verification of energy storage systems relies on efficient and accurate testing methods. As the key equipment to simulate real working conditions, the load bank's application strategy directly affects the test cycle and data reliability. Combined with industry practice, this paper deeply analyzes the technical path and typical cases to improve the test efficiency of energy storage system.
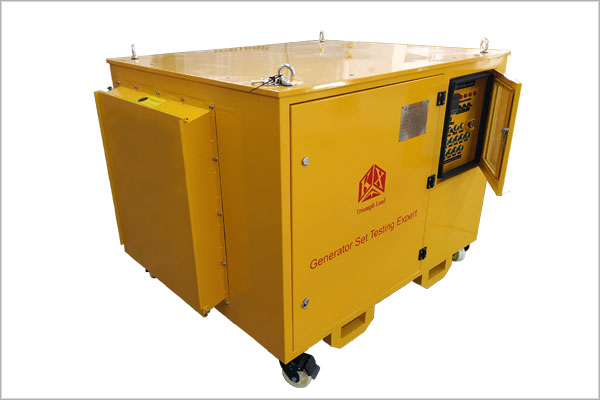
500kW Dummy Load Bank
First, energy storage test pain points and load bank core value
1. Test challenge analysis
Multi-scenario requirements: Complex conditions such as power grid fluctuations, extreme temperatures, and high-frequency charge and discharge need to be simulated.
Long cycle validation: Traditional cycle life testing takes 6-12 months, severely restricting development progress.
Safety requirements: need to accurately capture thermal runaway, overcharge/overdischarge and other critical states.
2. The key role of the load bank
Dynamic load simulation: millisecond response to load sudden changes (such as 0-100% SOC jump), reproduce the frequency modulation scenario of the power grid.
Energy closed loop management: Through the feedback type load bank to achieve charge and discharge energy recycling, reduce test costs.
Multi-parameter synchronous acquisition: synchronous recording of voltage, current, temperature, internal resistance and other 20 + key parameters, support failure analysis.
Two, three technical paths to improve test efficiency
1. Accelerated life test technology
1.1 Stress strengthening test method
Technical principle: By superimposing high temperature (60℃), high rate (3C charge and discharge) and other stress conditions, the test cycle is shortened to 3 months.
Case: A lithium battery manufacturer uses a load bank for accelerated life testing and finds that the capacity decay rate of the positive electrode material after 300 cycles is 40% faster than that of conventional testing, optimizing the formula in advance.
1.2 AI prediction model
Algorithm application: Capacity decay prediction model is established based on LSTM neural network, and 2000 lifetime can be predicted by inputting 100 cycles of data.
Verification data: The prediction error is controlled within ±5%, so an energy storage system company reduces the test sample size by 60%.
2. Construction of automated test platform
2.1 Full process automation
System architecture: Load Bank, BMS and environment box are linked by Modbus TCP protocol to achieve "working condition definition → test execution → data analysis" with one click.
Increased efficiency: After an energy storage integrator deployed an automation platform, the test time of a single system was reduced from 72 hours to 24 hours.
2.2 Standardization of fault injection
Predefined fault library: includes 12 types of fault modes such as overvoltage, undervoltage, and short circuit, and the reuse rate of test scripts reaches 80%.
Typical scenario: An energy storage power station injected grid disturbance faults in batches through load boxes before grid-connection, and completed 500 + fault response tests within 3 days.
3. Energy feedback technology innovation
3.1 Bidirectional converter integration
Technical breakthrough: The four-quadrant converter is used to achieve charge and discharge efficiency ≥95% and support AC/DC hybrid test.
Economic analysis: A light storage integration project uses a feedback load bank, saving electricity costs more than 800,000 yuan a year.
3.2 Grid friendly test
Function implementation: Simulate power grid frequency fluctuation (47-52Hz), voltage drop (0-100%), verify the energy storage system low voltage crossing ability.
Standard compliance: Help enterprises pass IEEE 1547, GB/T 36547 and other grid-connected certification tests.
3. Optimization plan for typical application scenarios
1. Test the battery pack consistency
Test method: Constant discharge of each single battery is carried out through the load bank, and inconsistent batteries with voltage difference > 5mV are screened.
Efficiency improvement: A car company uses a matrix load bank, and the test time of a single batch of 1000 batteries is compressed from 48 hours to 6 hours.
2. PCS (Energy Storage converter) test
Dynamic response test: the load bank completes the active/reactive power switch within 10ms to verify the power grid support capability of PCS.
Case: A PCS manufacturer completed 500 dynamic response tests through the load bank and found that 2% of the equipment had a response delay exceeding the standard.
3. Verify the thermal management system
Multi-physical field coupling test: load bank combined with thermal imager, synchronous monitoring of battery temperature field distribution and load current changes.
Optimization results: A battery thermal management scheme passed the test and reduced the temperature difference of the battery from 8℃ to 3℃.
Fourth, industry misunderstandings and solutions
1. Mistake 1: Excessive pursuit of test accuracy at the expense of efficiency
Risk: In order to achieve 0.01% current accuracy, a laboratory set the sampling frequency to 1MHz, resulting in a 10x increase in data storage.
Countermeasures: According to the standard requirements to select the appropriate accuracy (such as IEC 62619 provisions of 0.1% FS), the use of adaptive sampling technology.
2. Mistake 2: Ignoring the synchronization of environmental parameters
Typical problem: the heating rate of the environment chamber lags behind the power change of the load chamber, resulting in mismatch of the temperature-load curve.
Solution: PID linkage control is adopted to ensure that the time difference between temperature and load change is less than 3 seconds.
5. Future technology trends
1. Test the digital twin driver
Technical application: Build the battery digital twin model based on the measured data of the load bank to predict the failure mode in advance.
Case study: A research institution has reduced the development cycle of new batteries by 50% through digital twin technology.
2. Edge computing localization analysis
Architecture innovation: Edge computing module is installed in the load bank to analyze test data in real time and output decision suggestions.
Advantages: Reduce cloud data transmission by 90%, suitable for field testing of energy storage projects in remote areas.